why should I make a copy of a data frame in pandas
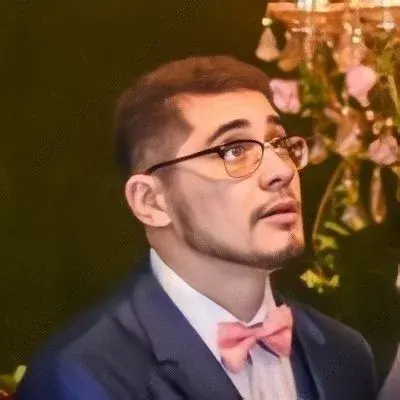
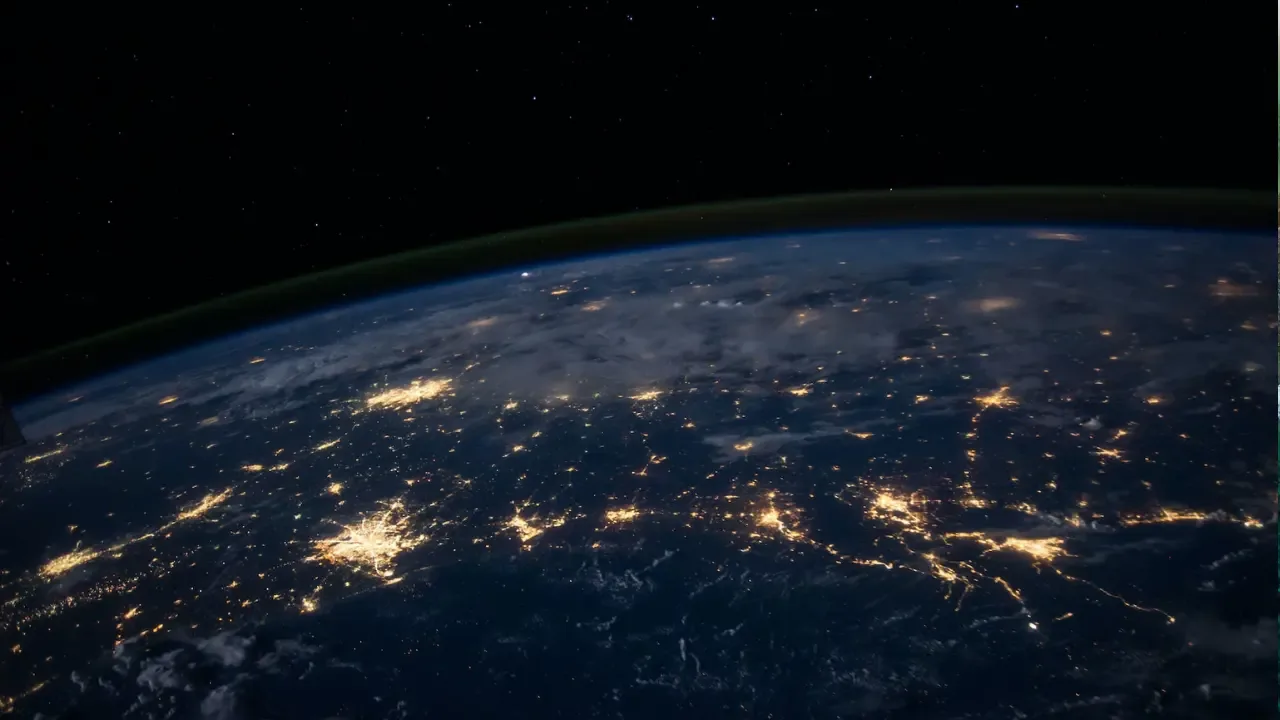
📝Why Should I Make a Copy of a Data Frame in Pandas?
Have you ever wondered why some programmers make a copy of a DataFrame using the .copy()
method in Pandas? 🤔 In this blog post, we will address this common question and explore the reasons behind making a copy. By the end, you'll have a clear understanding of why making a copy is essential and what happens if you don't. So let's dive in! 💪
🧐 The Problem
When selecting a sub DataFrame from a parent DataFrame, some programmers opt to make a copy of the data frame using the .copy()
method, like this:
X = my_dataframe[features_list].copy()
Rather than simply assigning it without the .copy()
method, like this:
X = my_dataframe[features_list]
But why do they do this? 🤷♀️ What's the difference?
💡 The Solution
The reason programmers make a copy of a DataFrame is to avoid potential issues with data contamination. Let me explain with an example 👇
Assume we have a DataFrame my_dataframe
, which contains various columns: A
, B
, and C
. We want to create a new DataFrame X
that only includes the columns A
and B
.
Without making a copy:
X = my_dataframe[["A", "B"]]
If you make changes to X
, such as modifying values or dropping rows, these changes will also be reflected in the original DataFrame my_dataframe
. This is because both X
and my_dataframe
are referring to the same memory location.
On the other hand, by making a copy:
X = my_dataframe[["A", "B"]].copy()
Any changes you make to X
will not affect the original DataFrame my_dataframe
. This is because X
is an entirely separate object, stored in a different memory location.
⚠️ The Consequences of Not Making a Copy
If you do not make a copy and inadvertently modify the sub DataFrame, you risk altering the original data unintentionally. This can lead to inaccurate analysis or even data loss. 😱
For instance, imagine you're working on a machine learning project and you accidentally overwrite your training data while making transformations to a sub DataFrame. The consequences could be disastrous! 😨
Thus, making a copy acts as a safeguard. It ensures that any changes made to the sub DataFrame do not impact the original data, keeping your analysis intact and preventing unwanted surprises.
📢 Final Thoughts
Now that you understand the importance of making a copy of a DataFrame in Pandas, you should always consider using .copy()
when working with sub DataFrames. This simple practice can save you from potential data contamination risks and help maintain a clean and reliable data analysis workflow. 💯
So next time you're creating a sub DataFrame, don't forget to make a copy and keep your original data safe and sound! Happy coding! 😊
I hope you found this blog post insightful and helpful. If you have any questions or suggestions, feel free to leave a comment below. Let's keep the discussion going! 👇👇👇
Take Your Tech Career to the Next Level
Our application tracking tool helps you manage your job search effectively. Stay organized, track your progress, and land your dream tech job faster.
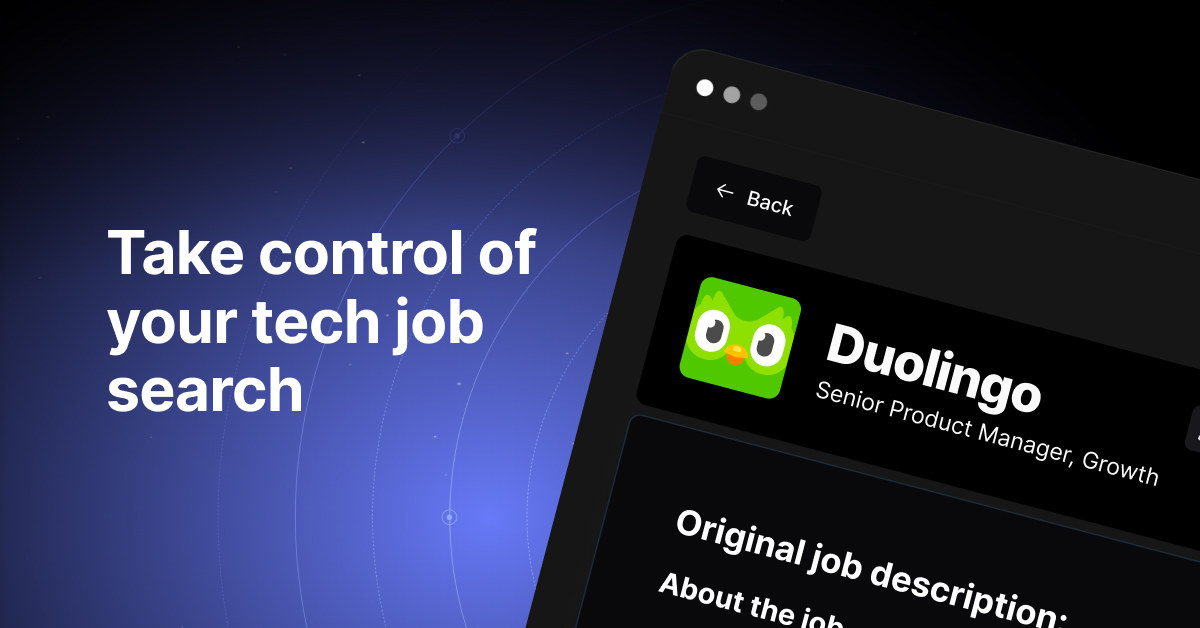